A recurring challenge I hear about in my discussions with AI professionals is a lapse in data governance. As companies evaluate and deploy AI-enabled applications, they too often overlook the basics of data governance.
Simply put, data governance is the foundation for responsible and ethical AI development and implementation.
Last week, I came across another instance of an organization stumbling into a significant privacy and security lapse. The organization in question was a correctional facility working with an AI developer to implement a pilot project for an element of their ERP system. While the specific use case isn’t the focus here, the primary issue lies in the pilot project’s AI model being trained on sensitive data without proper attention to privacy and security. During the validation phase, it became apparent that the AI model was exposing extremely sensitive data.
In short, sensitive information about corrections officers, inmates, visitors, and specific incidents was readily accessible to anyone using the AI-enabled application. Although the exposure was limited to developers, testers, and internal employees, the exposure itself represents a failure in both data governance and AI governance.
The gap in data governance was due to a failure to inventory, classify, and manage the data according to its sensitivity and established policies. Even if some governance mechanisms, such as policies and procedures, were in place, they failed to prevent the exposure risk when the AI model developers ingested the data.
The responsibility for this oversight lies primarily with the correctional facility.
On the AI governance side, the failure was in not identifying the sensitivity of the data and not implementing privacy and security measures by design. Detecting the data exposure during the testing phase is a costly mistake. Correcting this will likely require re-implementing baseline data governance practices, integrating privacy-enhancing technologies, and retraining the model.
The AI model development company bears responsibility for this oversight.
Remarkably, this type of data and AI governance failure is all too common. I’ve heard similar stories about companies inadvertently exposing sensitive HR data, such as salary information, and PHI within healthcare organizations. One of my colleagues at Microsoft put it simply:
“If your organization has any hidden or sensitive files, AI will uncover them.”
Take aways:
- For all the companies out there developing your AI strategy, don’t forget to include data and AI governance in your plans, and insist upon it with your suppliers.
- For AI developers and AI application companies, incorporate AI governance into your engagement model with your clients.
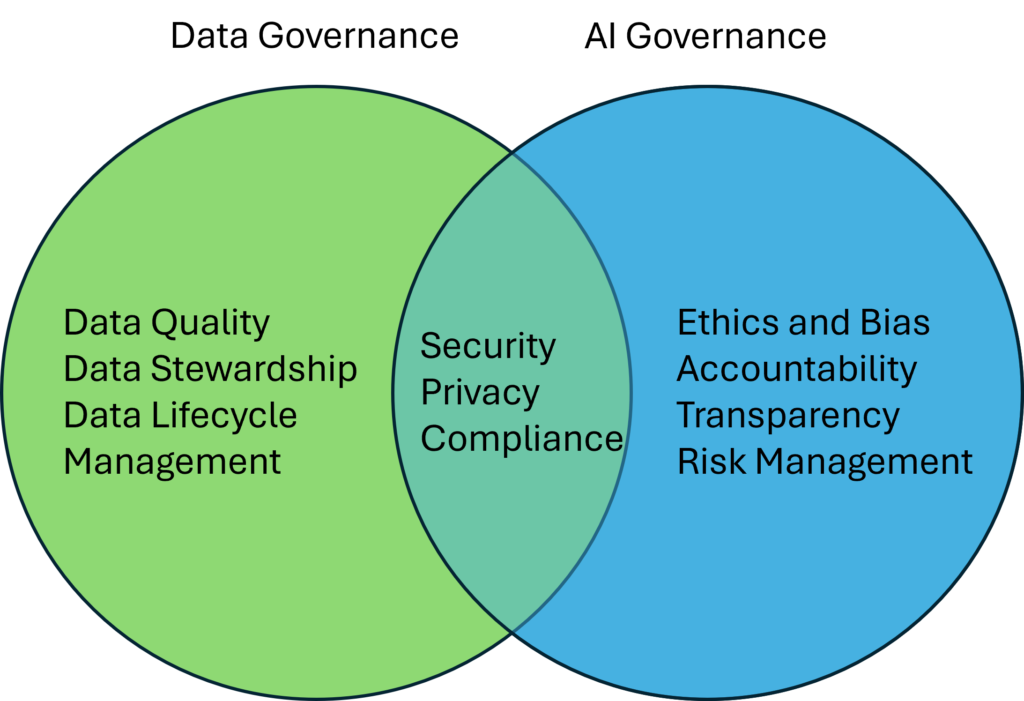